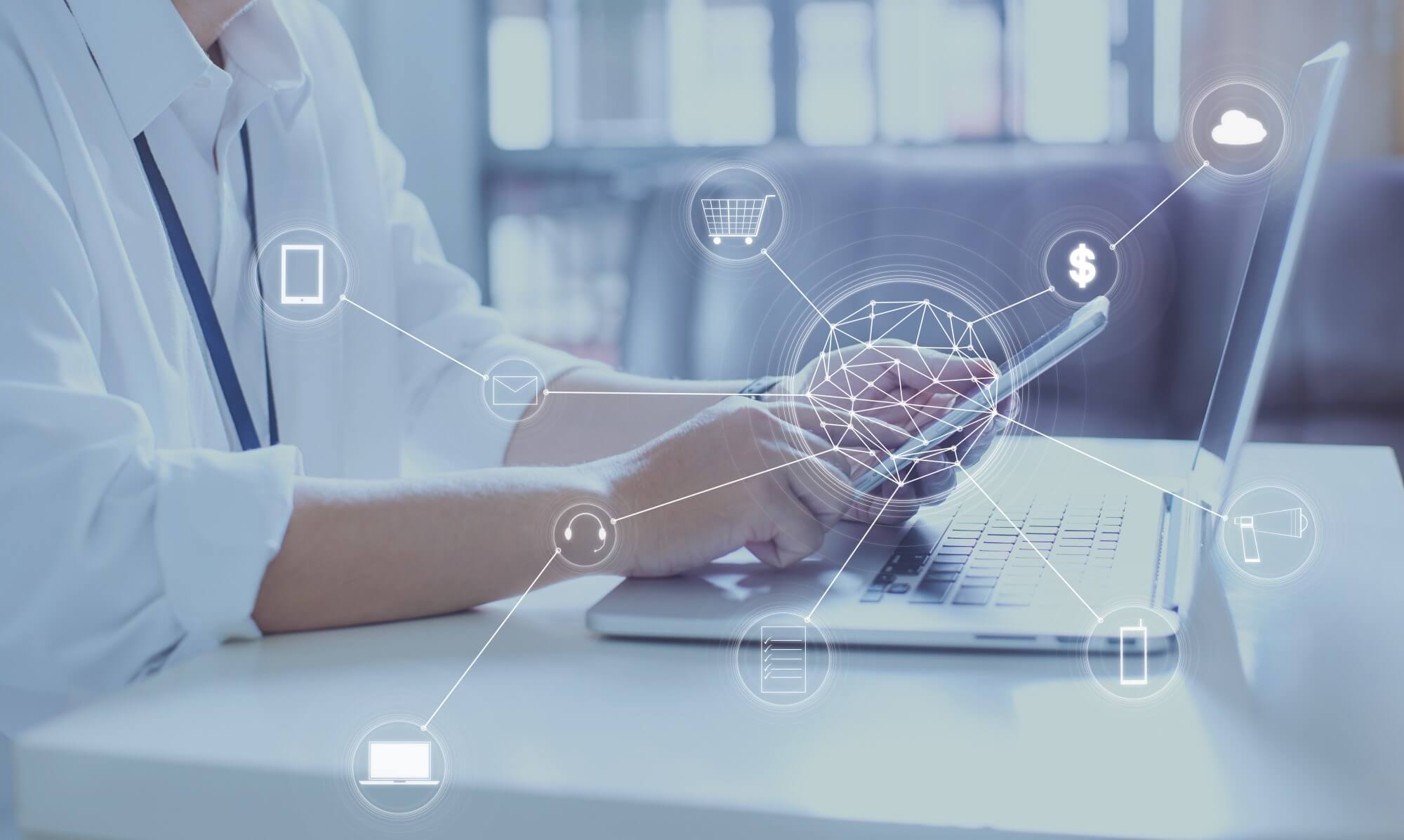
The demand for data lakes is growing. Fast.
The data lake market will generate revenues of more than $86 billion by 2032, driven in part by IoT-dependent verticals like manufacturing, healthcare, and retail, according to Polaris Market Research. A data lake is a centralized repository for storing all your raw data, regardless of source, so you can combine it, visualize it, and even query it. It is essential for any organization wanting to take advantage of generative AI, now or in the future. But if you’re planning to implement one—or just want to get your data out of silos and into the cloud—it’s important to get it right.
At Kopius, we have helped a fair number of companies whose first try at setting up a data lake didn’t yield the kind of results they were looking for to get projects back on track. Given that risk, it’s no surprise that we also talk with companies that are wondering if it’s worth it.
The answer is a resounding yes.
When properly implemented, putting your data in a data lake or similar environment will enable you to better meet customer needs, solve business problems, get products to market faster, more closely manage your supply chain, and even unearth insights about your business that a human might not even be able to see.
And setting one up doesn’t have to be a long, arduous journey—think of it as more of a quick trip.
Data Lakes Deliver Strategic Advantages: Insights at Speed
Whether you’re moving your data from an internal SQL or other server to Cloud or already have your data in one or more disparate cloud applications, putting all that raw data into a data lake has strategic advantages. Chief among them, and the one that is top of mind for many organizations, is that it is an essential first step in preparing yourself to take advantage of generative AI, (GenAI), which requires raw data in a modern environment.
Another big advantage of data lakes is simply speed. Once all your data is in a data lake, you can set up pipelines to ingest and structure it. You don’t have to go through the tedious process of standardizing or normalizing it to build dashboards or reports or do whatever you need to do. It’s a much faster process than your old SQL server or whatever solution you’re using now. For example, a global healthcare consulting and services company we worked with spent months coding a pipeline to ingest data they needed for a process that took three to four hours to run. Once we implemented their data lake, we set up a couple of pipelines in just two weeks to support the activities they were already doing, with processing time of just minutes.
As fast as businesses move today, all that speed gives you a competitive advantage.
A Data Lake Isn’t the Endgame
Implementing a data lake isn’t the end game—it’s a starting point. It’s just one of several critical components in an overarching, long-term data strategy. Data must be structured—formatted so you can visualize it, query it, or do whatever it is you need to do. So even though it’s somewhat straightforward to stand up a proof of concept, it’s important to know what your endgame is. You’ll need to have some big picture idea of how you want to use your data, because that informs what solution set is best for you. And the market for solutions is both somewhat nascent and already very complex.Fortunately, at Kopius, we have a process for walking you through all these important considerations to find a point of departure or move you further along the data maturity path, including helping you narrow down what your endgame is. It’s designed to get you on the right path up front, so you get the outcomes you are looking for.
Jumpstart Your Data Lake Initiative with a Proof of Concept
At Kopius, we harness the power of people, data, and emerging technologies to build innovative data lake solutions that help our customers navigate continual change and solve formidable challenges. To accelerate our customers’ success, we’ve designed a JumpStart program to prioritize digital transformation together.
Related Services:
Additional Resources:
- Data Lakes: The Foundation of Big Data Analytics
- Comprehensive Guide to Integrating Diverse Data Sources
- Building a Data-Driven Culture