by Yuri Brigance
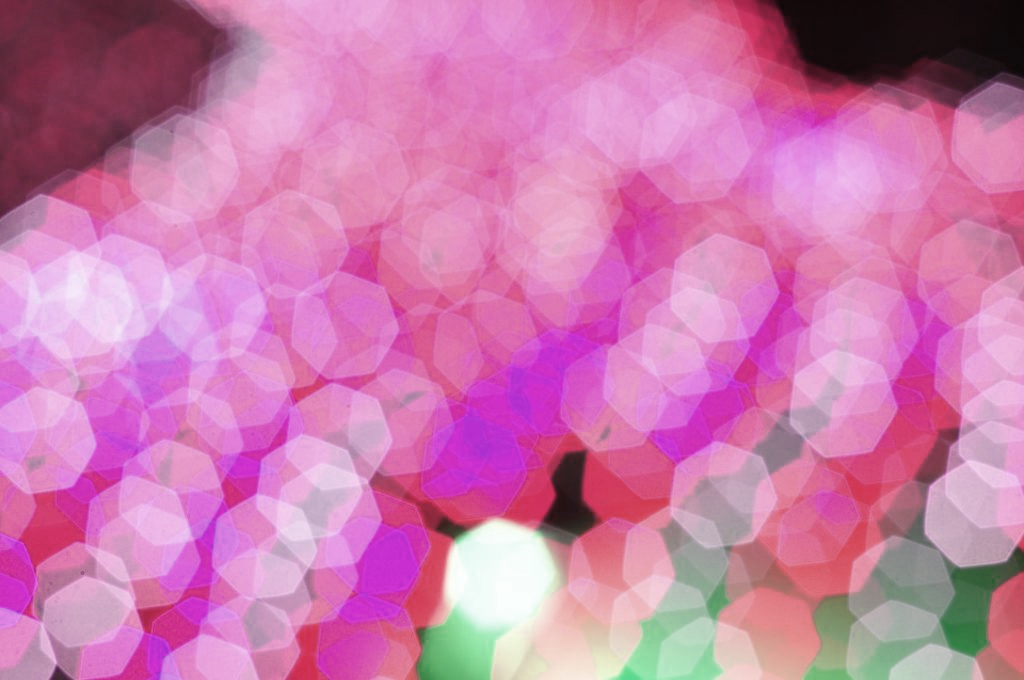
I previously wrote about deep learning at the Edge. In this post I’m going to describe the process of setting up an end-to-end Machine Learning (ML) workflow for different types of machine learning.
There are three common types of machine learning training approaches, which we will review here:
- Supervised
- Unsupervised
- Reinforcement
And since all learning approaches require some type of training data, I will also share three methods to build out your training dataset via:
- Human Annotation
- Machine Annotation
- Synthesis / Simulation
Supervised Learning:
Supervised learning uses a labeled training set of both inputs and outputs to teach a model to yield the desired outcome. This approach typically relies on a loss function, which is used to evaluate training accuracy until the error has been sufficiently minimized.
This type of learning approach is arguably the most common, and in a way, it mimics how a teacher explains the subject matter to a student through examples and repetition.
One downside to supervised learning is that this approach requires large amounts of accurately labeled training data. This training data can be annotated manually (by humans), via machine annotation (annotated by other models or algorithms), or completely synthetic (ex: rendered images or simulated telemetry). Each approach has its pros and cons, and they can be combined as needed.
Unsupervised Learning:
Unlike supervised learning, where a teacher explains a concept or defines an object, unsupervised learning gives the machine the latitude to develop understanding on its own. Often with unsupervised learning, the machines can find trends and patterns that a person would otherwise miss. Frequently these correlations elude common human intuition and can be described as non-semantic. For this reason, the term “black box” is commonly applied to such models, such as the awe-inspiring GPT-3.
With unsupervised learning, we give data to the machine learning model that is unlabeled and unstructured. The computer then identifies clusters of similar data or patterns in the data. The computer might not find the same patterns or clusters that we expected, as it learns to recognize the clusters and patterns on its own. In many cases, being unrestricted by our preconceived notions can reveal unexpected results and opportunities.
Reinforcement Learning:
Reinforcement learning teaches a machine to act in a semi-supervised approach. The machines are rewarded for correct answers, and the machine wants to be rewarded as much as possible. Reinforcement learning is an efficient way to train a machine to learn a complicated task, such as playing video games or teaching a legged robot to walk.
The machine is motivated to be rewarded, but the machine doesn’t share the operator’s goals. So if the machine can find a way to “game the system” and get more reward at the cost of accuracy, it will greedily do so. Just as machines can find patterns that humans miss in unsupervised learning, machines can also find missed patterns in reinforcement learning, and exploit those invisible patterns to receive additional reinforcement. This is why your experiment needs to be airtight to minimize exploitation by the machines.
For example, an AI twitterbot that was trained with reinforcement learning was rewarded for maximizing engagement. The twitterbot learned that engagement was extremely high when it posted about Hitler.
This machine behavior isn’t always a problem – for example reinforcement learning helps machines find bugs in video games that can be exploited if they aren’t resolved.
Datasets:
Machine Learning implies that you have data to learn from. The quality and quantity of your training data has a lot to do with how well your algorithm can perform. A training dataset typically consists of samples, or observations. Each training sample can be an image, audio clip, text snippet, sequence of historical records, or any other type of structured data. Depending on which machine learning approach you take, each sample may also include annotations (correct outputs / solutions) that are used to teach the model and verify the results. Training datasets are commonly split into groups where the model only trains on a sub-set of all available data. This allows a portion of the dataset to be used for validation of the model, to ensure that the model has generalized enough data to perform well on data it has not seen before.
Regardless of which training approach you take, your model can be prone to bias which may be inadvertently introduced through unbalanced training data, or selection of the wrong inputs. One example is an AI criminal risk assessment tool used by courts to evaluate how likely a defendant is to reoffend based on their profile as input. Because the model was trained on historical data, which included years of disproportionate targeting by law enforcement of low-income and minority groups, the resulting model produced higher risk scores for low-income and minority individuals. It is important to remember that most machine learning models pick up on statistical correlations, and not necessarily causations.
Therefore, it is highly desirable to have a large and balanced training dataset for your algorithm, which is not always readily available or easy to obtain. This is a task which may initially be overlooked by businesses excited to apply machine learning to their use cases. Dataset acquisition is as important as the model architecture itself.
One way to ensure that the training dataset is balanced is through Design of Experiments (DOE) approach, where controlled experiments are planned and analyzed to evaluate the factors which control the value of an output parameter or group of parameters. DOE allows for multiple input factors to be manipulated, determining their effect on the model’s response. Thus, giving us the ability to exclude certain inputs which may lead to biased results, as well as gain a better understanding of the complex interactions that occur inside the model.
Here are three examples of how training data is collected, and in some cases generated:
- Human Labeled Data:
What we refer to human labeled data is anything that has been annotated by a living human, either through crowdsourcing or by querying a database and organizing the dataset. An example of this could be annotating facial landmarks around the eyes, nose, and mouth. These annotations are pretty good, but in certain instances can be imprecise. For example, the definition of “the tip of the nose” can be interpreted differently by different humans who are tasked with labeling the dataset. Even simple tasks, like drawing a bounding box around apples in photos can have “noise” because the bounding box may have more or less padding, may be slightly off center, and so on.
Human labeled data is a great start if you have it. But hiring human annotators can be expensive and prone to error. Various services and tools exist, from AWS SageMaker GroundTruth to several startups which make the labeling job easier for the annotators, and also connect annotation vendors with clients.
It might be possible to find an existing dataset in the public domain. In an example with facial landmarks, we have WFLW, iBUG, and other publicly available datasets which are perfectly suitable for training. Many have licenses that allow commercial use. It’s a good idea to research whether someone has already produced a dataset that fits your needs, and it might be worth paying for a small dataset to bootstrap your learning process.
2. Machine Annotation:
In plain terms, machine annotation is where you take an existing algorithm or build a new algorithm to add annotations to your raw data automatically. It sounds like a chicken and egg situation, but it’s more feasible than it initially seems.
For example, you might already have a partially labeled dataset. Let’s imagine you are labeling flowers in bouquet photos, and you want to identify each flower. Maybe you had some portion of these images already annotated with tulips, sunflowers, and daffodils. But there are still images in the training dataset that contain tulips which have not been annotated, and new images keep coming in from your photographers.
So, what can you do? In this case, you can take all the existing images where the tulips have already been annotated and train a simple tulip-only detector model. Once this model reaches sufficient accuracy, you can fill in the remaining missing tulip annotations automatically. You can keep doing this for the other flowers. In fact, you can crowdsource humans to annotate just a small batch of images with a specific new flower, and that should be enough to build a dedicated detector that can machine-annotate your remaining samples. In this way, you save time and money by not having humans annotate every single image in your training set or every new raw image that comes in. The resulting dataset can be used to train a more complete production-grade detector, which can detect all the different types of flowers. Machine annotation also gives you the ability to continue improving your production model by continuously and automatically annotating new raw data as it arrives. This achieves a closed-loop continuous training and improvement cycle.
Another example is where you have incompatible annotations. For example, you might want to detect 3D positions of rectangular boxes from webcam images, but all you have are 2D landmarks for the visible box corners. How do you estimate and annotate the occluded corners of each box, let alone figure out their position in 3D space? Well, you can use a Principal Component Analysis (PCA) morphable model of a box and fit it to 2D landmarks, then de-project the detected 3D shape into 3D space using camera intrinsics . This gives you full 3D annotations, including the occluded corners. Now you can train a model that does not require PCA fitting.
In many cases you can put together a conventional deterministic algorithm to annotate your images. Sure, such algorithms might be too slow to run in real-time, but that’s not the point. The point is to label your raw data so you can train a model, which can be inferenced in milliseconds.
Machine annotation is an excellent choice to build up a huge training dataset quickly, especially if your data is already partially labeled. However, just like with human annotations, machine annotation can introduce errors and noise. Carefully consider which annotations should be thrown out based on a confidence metric or some human review, for example. Even if you include a few bad samples, the model will likely generalize successfully with a large enough training set, and bad samples can be filtered out over time.
3. Synthetic Data
With synthetic data, machines are trained on renderings or in hyper-realistic simulations – think of a video game of a city commute, for example. For Computer Vision applications, a lot of synthetic data is produced via rendering, whether you are rendering people, cars, entire scenes, or individual objects. Rendered 3D objects can be placed in a variety of simulated environments to approximate the desired use case. We’re not limited to renderings either, as it is possible to produce synthetic data for numeric simulations where the behavior of individual variables is well known. For example, modeling fluid dynamics or nuclear fusion is extremely computationally intensive, but the rules are well understood – they are the laws of physics. So, if we want to approximate fluid dynamics or plasma interactions quickly, we might first produce simulated data using classical computing, then feed this data into a machine learning model to speed up prediction via ML inference.
There are vast examples of commercial applications of synthetic data. For example, what if we needed to annotate the purchase receipts for a global retailer, starting with unprocessed scans of paper receipts? Without any existing metadata, we would need humans to manually review and annotate thousands of receipt images to assess buyer intentions and semantic meaning. With a synthetic data generator, we can parameterize the variations of a receipt and accurately render them to produce synthetic images with full annotations. If we find that our model is not performing well under a particular scenario, we can just render more samples as needed to fill in the gaps and re-train.
Another real-world example is in manufacturing where “pick-and-place” robots use computer vision on an assembly line to pack or arrange and assemble products and components. Synthetic data can be applied in this scenario because we can use the same 3D models that were used to create injection molds of the various components to make renderings as training samples that teach the machines. You can easily render thousands of variations of such objects being flipped and rotated, as well as simulate different lighting conditions. The synthetic annotations will always be 100% precise.
Aside from rendering, another approach is to use Generative Adversarial Network (GAN) generated imagery to create variation in the dataset. Training GAN models usually requires a decent number of raw samples. With a fully trained GAN autoencoder it is possible to explore the latent space and tweak parameters to create additional variation. Although it’s more complex than classical rendering engines, GANs are gaining steam and have their place in the synthetic data generation realm. Just look at these generated portraits of fake cats!
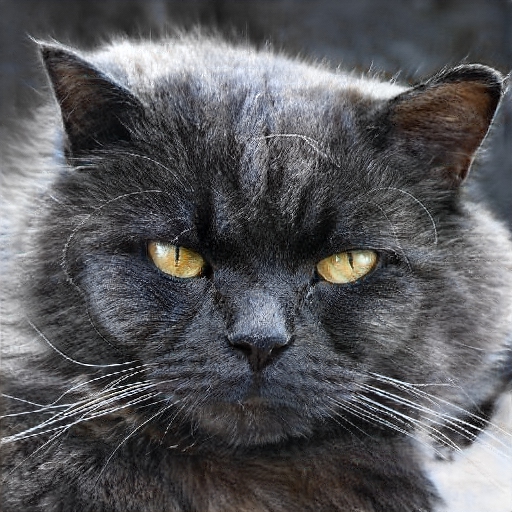
Choosing the right approach:
Machine learning is on the rise across industries and in businesses of all sizes. Depending on the type of data, the quantity, and how it is stored and structured, Valence can recommend a path forward which might use a combination of the data generation and training approaches outlined in this post. The order in which these approaches are applied varies by project, and boils down to roughly four phases:
- Bootstrapping your training process. This includes gathering or generating initial training data and developing a model architecture and training approach. Some statistical analysis (DOE) may be involved to determine the best inputs to produce the desired outputs and predictions.
- Building out the training infrastructure. Access to Graphics Processing Unit (GPU) compute in the cloud can be expensive. While some models can be trained on local hardware at the beginning of the project, long-term a scalable and serverless training infrastructure and proper ML experiment lifecycle management strategy is desirable.
- Running experiments. In this phase we begin training the model, adjusting the dataset, experimenting with the model architecture and hyperparameters. We will collect lots of experiment metrics to gauge improvement.
- Inference infrastructure. This includes integrating the trained model into your system and putting it to work. This can be cloud-based inference, in which case we’ll pick the best serverless approach that minimizes cloud expenses while maximizing throughput and stability. It might also be edge inference, in which case we may need to optimize the model to run on a low-powered edge CPU, GPU, TPU, VPU, FPGA, or a combination of thereof.
What I wish every reader understood is that these models are simple in their sophistication. There is a discovery process at the onset of every project where we identify the training data needs and which model architecture and training approach will get the desired result. It sounds relatively straight forward to unleash a neural network on a large amount of data, but there are many details to consider when setting up Machine Learning workflows. Just like real-world physical research, Machine Learning requires us to up a “digital lab” which contains the necessary tools and raw materials to investigate hypotheses and evaluate outcomes – which is why we call AI training runs “experiments”. Machine Learning has such an array of truly incredible applications that there is likely a place for it in your organization as part of your digital journey.
Additional resources: