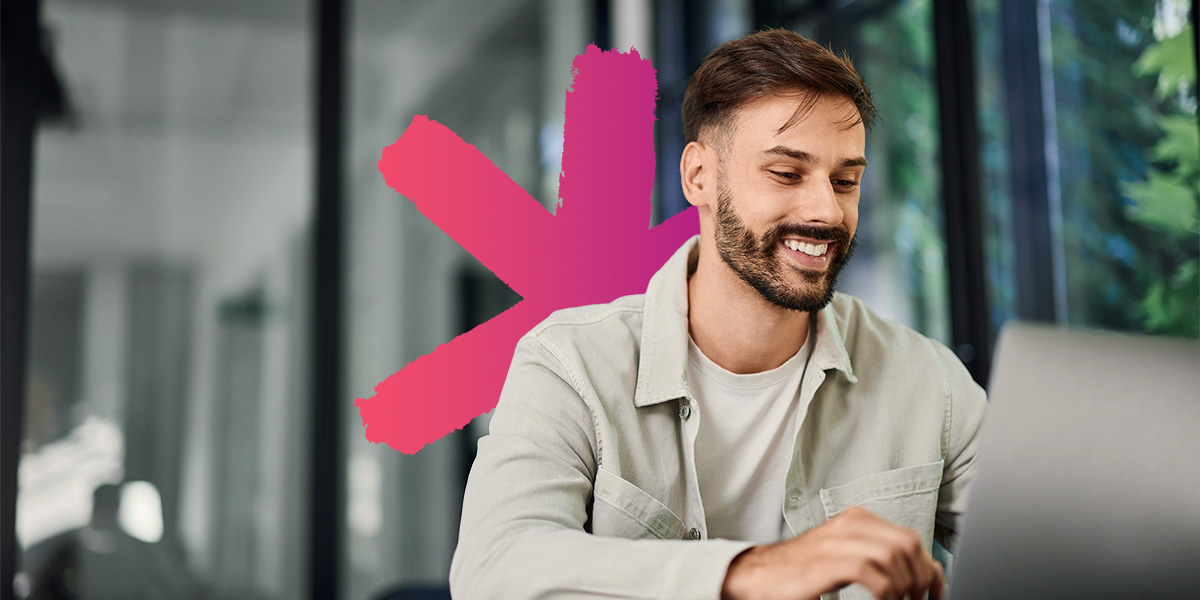
Nowadays, data is everything. It fuels your decisions, drives business growth, and improves customer relationships. Data governance and regulatory compliance are heavily intertwined aspects of managing and securing your organization’s data. A strong data governance policy sets the standard for how you collect, store, process, access, and use data throughout its life cycle.
Without a proper governance strategy, it becomes increasingly difficult to maintain compliance when handling and processing sensitive data, such as financial, personal, or health records. Failure to comply with these regulations can result in significant financial and reputational losses for your business. Understanding data governance and compliance is key to implementing robust policies and practices.
Understanding Data Governance and Regulatory Compliance
The terms “data governance” and “regulatory compliance” are often used interchangeably, but they differ. Before you can implement effective data governance, it’s important to know the definitions, objectives, and importance of each term.
What Is Data Governance?
Data governance refers to the processes, guidelines, and rules that outline how an organization manages its resources, including data. These guidelines exist to make sure data is accessible, accurate, consistent, and secure. The key components of data governance typically include:
- Ensuring regulatory compliance.
- Maintaining data quality.
- Outlining roles and responsibilities.
- Monitoring the use of resources.
- Facilitating data integration and interoperability.
- Scaling based on demand.
- Securing sensitive data against unauthorized access and breaches.
- Improving cost-effectiveness.
Data governance is essential for protecting and maintaining crucial data and confirming that it aligns with business objectives. Data governance also plays a pivotal role in helping organizations meet regulatory compliance requirements for data management, privacy, and security. As regulations continue to evolve, so does the need to meet them. Data governance supports organizations in this regard by establishing and enforcing policies for responsible data use.
What Is Regulatory Compliance?
Regulatory compliance refers to the regulations, laws, and standards that an organization must meet within its industry. Compliance standards vary by state and industry, but their primary purpose is to ensure organizations securely handle personal and sensitive data. Data protection and privacy laws are essential aspects of regulatory compliance. For instance, health care organizations are required to meet industry-specific regulations like the Health Insurance Portability and Accountability Act to protect patient privacy.
The Fair Credit Reporting Act outlines protection measures for sensitive personal information regarding consumer credit report records. The Family Educational Rights and Privacy Act is another example of a data governance policy that protects access to students’ educational data. Compliance is essential for organizations because it enables them to build trust with their customers, improve their reputation, and avoid legal risks.
Data Governance vs. Compliance
Data governance refers to how organizations use, manage, and control their data internally, while regulatory compliance is about how they adhere to external regulations. Data governance guides decision-makers to be proactive, while compliance is often reactive.
Can an organization be compliant without data governance? The answer is yes. It’s possible for your organization to have data governance standards in place without being fully compliant if your policies do not meet industry or external regulations. Alternatively, your organization may be compliant by meeting the minimum regulatory standards without establishing an effective data governance framework.
While one is possible without the other, both data governance and compliance are crucial for a cohesive data management strategy. Governance builds the framework within which compliance operates to keep your business efficient. These two closely related aspects help your organization achieve business objectives, identify opportunities for strategic data utilization, and improve legal integrity.
The Role of Data Governance in Ensuring Compliance
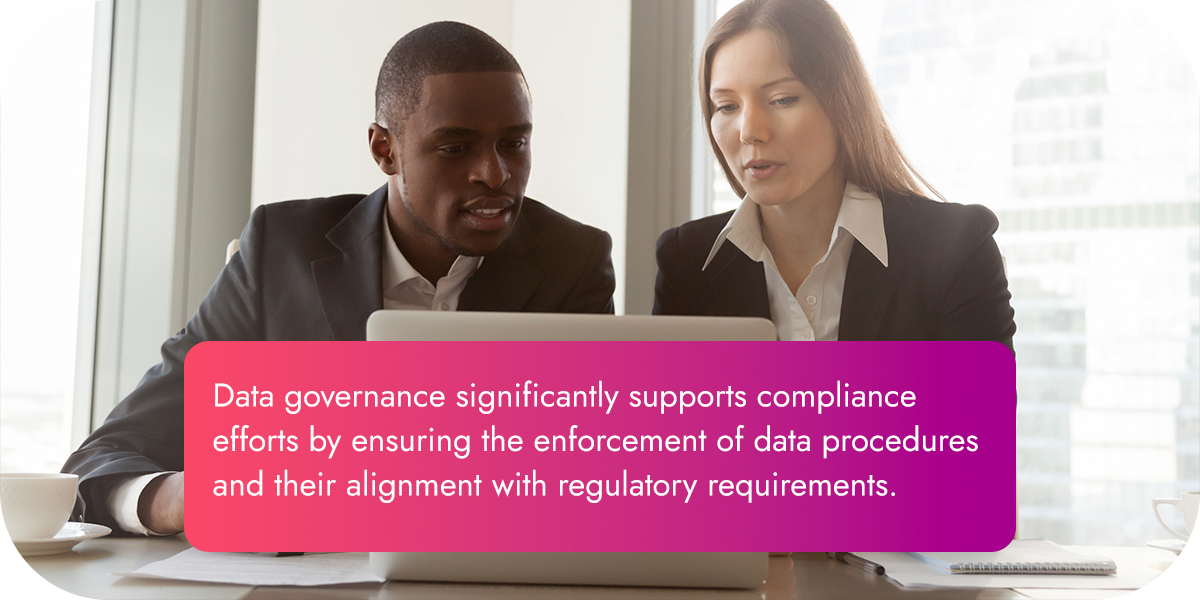
Now that you know the distinctions between data governance and compliance, it’s time to examine the integral role of data governance in adhering to policy, regulatory, and legal requirements.
Data governance significantly supports compliance efforts by ensuring the enforcement of data procedures and their alignment with regulatory requirements. Additionally, having strong data governance standards in place can help organizations achieve data compliance by:
- Simplifying the interpretation of compliance laws and regulations.
- Proactively addressing compliance needs.
- Establishing data stewards to create data governance consistency.
- Identifying data governance risks and areas of noncompliance.
- Reducing the complexity required to adhere to regulatory standards.
- Maintaining well-documented data processes to facilitate streamlined audits.
- Continuously monitoring data quality management practices.
- Establishing the traceability of data processes.
Similarly, poor data quality can lead to compliance issues, which can result in fines, penalties, and legal complications. As a result, data governance procedures are necessary to verify that data is ethically and securely aligned with industry regulations. Safeguarding your organizational data’s integrity with data governance policies can also enhance your ability to demonstrate compliance with external standards — a benefit to all stakeholders.
Challenges in Meeting Regulatory Compliance
What stands in the way of compliance? In the digital age, organizations in all industries face obstacles due to ever-changing regulatory landscapes. Here are some of the most common challenges in working toward compliance:
1. Evolving Regulations
Laws and regulations constantly change, making it challenging for organizations to keep up. As lawmakers develop new policies for protecting consumer data, organizations must frequently update to meet diverse compliance demands. Following the continuous growth of data governance regulations can put additional strain on compliance teams as they strive to safeguard data integrity.
2. Gaps and Overlaps
Alongside rapidly evolving laws is the challenge of balancing internal policies with external regulations. As new regulations arise to meet data privacy and security concerns, organizations must address existing gaps and overlaps to create consistency.
3. Monitoring Needs
Tracking data flow and usage is a key part of data governance. However, organizations that fail to properly monitor and audit data practices may struggle to adhere to compliance regulations. Some organizations may lack the staff or resources needed for continuous monitoring.
4. Vast Amounts of Data
It’s no secret that businesses are collecting, using, and storing more data than ever. Maintaining compliance becomes even more complex as more and more data flows in. Without proper data storage, managing these large volumes of data can be difficult.
5. Vulnerability of Legacy Systems
Relying on outdated technology to maintain compliance is nearly impossible due to the lack of security upgrades and other modern compliance essentials. Organizations that still use legacy systems will find it increasingly complex to meet today’s strict regulations.
6. Risk of Data Breaches
Data breaches increased by 20% in 2023, along with significant spikes in ransomware attacks and theft of personal data. However, as companies put more and more of their data into computerized systems, the risk of data breaches grows without proper configuration and security measures.
7. Lack of Expertise
As a result of increased data security concerns, there is a growing need for skilled personnel who can navigate the legal aspects of data compliance. Staff training is also required to keep employees up to date on changing regulations to ensure ongoing compliance.
8. Cost Concerns
Maintaining compliance can be costly, especially when factoring in hiring skilled personnel, training internal compliance staff, and upgrading technology. Maintaining ongoing compliance in an evolving landscape of regulations can lead to increased operating costs as continuous audits and assessments are needed.
Benefits of Implementing Data Governance Strategies for Compliance
Implementing a robust data governance framework is essential to creating a culture of data compliance. Here are some advantages you can expect with data governance policies:
1. Minimized Legal Risks
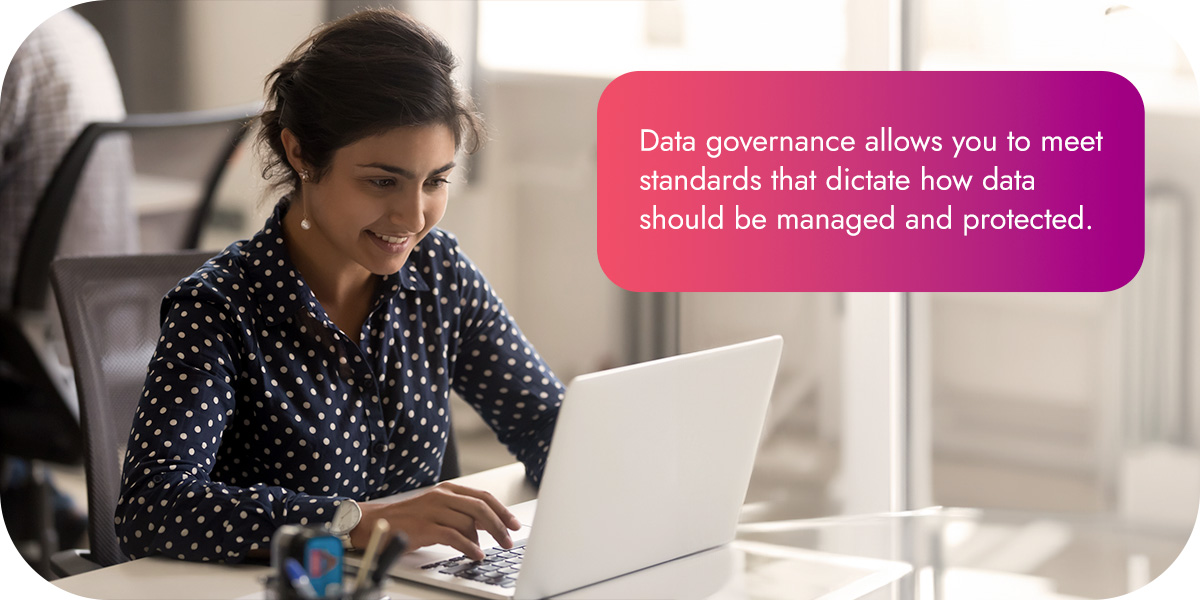
Data governance procedures can help your organization identify and manage potential compliance risks. Adhering to data regulations can protect your organization from legal consequences, such as fines and penalties. Without an organized framework for every team member to follow, it can be challenging to know whether you’re meeting regulatory requirements.
Data governance allows you to meet standards that dictate how data should be managed and protected. Similarly, data governance guidelines can simplify compliance reporting and audits, which can also reduce the risk of fines and legal issues.
2. Enhanced Security
Robust data security measures can benefit businesses across all industries. Establishing data governance strategies can protect sensitive data from breaches and cyber threats. Data governance also prevents the unauthorized use or misuse of data, which is particularly important in the health care and finance industries. In today’s landscape of increasing cybersecurity hacks and threats, data governance allows for a proactive approach to organizational security.
3. Improved Decision-Making
Data governance is a powerful tool that decision-makers across your organization can utilize to drive your business forward. Data governance strategies can help your teams make well-informed decisions by gathering key insights on how data is being accessed, handled, and secured.
4. Increased Data Accessibility and Quality
Effective data governance strategies help your teams properly manage your data, meaning it will be organized and cataloged effectively. As a result, users can find the data they need when they need it and expect it to be accurate, up to date, and complete. Additionally, you and your teams won’t have to rely on poor-quality data to make important decisions.
Adhering to data regulations can lead to minimal errors and allow employees to quickly and easily access the information they need to do their jobs. Organizations that have multiple business partners or units can feel confident in data sharing, knowing their data is consistent and well-controlled.
5. Improved Compliance
Though the existence of data governance strategies does not make an organization inherently more compliant, it creates an environment that prioritizes compliance. Establishing data governance strategies demonstrates that organizations take data privacy seriously and will continue to update policies as needed to align with relevant regulations. Companies that use data governance procedures may also be more likely to meet regulations that govern the use and protection of data because they’re well-informed of the potential risks of noncompliance.
6. Strengthened Reputation
Transparency is key when it comes to building and maintaining customer relationships. Organizations that adhere to data regulations and strive to keep consumer data safe may enhance their reputation among stakeholders, customers, partners, and employees. They are more likely to foster trust among clients and consumers who want to know that their data is being handled responsibly.
7. Facilitate Room for Innovation
When it comes to data, organizations have to think three steps ahead. Data governance strategies ensure your data is well-managed and maintained, creating an environment conducive to business innovation. Employees can access high-quality data faster, enabling more time for innovative solutions and new ideas. What’s more, a robust data governance framework signifies to stakeholders that future innovation efforts are built on secure, dependable data governance practices.
8. Identify New Revenue Opportunities
Taking a proactive approach to data security with data governance allows you to identify potential risks and gaps in your current workflow. However, it can also help you identify opportunities for revenue growth.
Effective data governance means you can more easily view customer trends and market insights that enable you to develop new products and services to meet current demands. Data governance procedures turn your data into a strategic asset, allowing you to take advantage of opportunities to improve sales and customer satisfaction.
Implementing a Data Governance Framework for Compliance
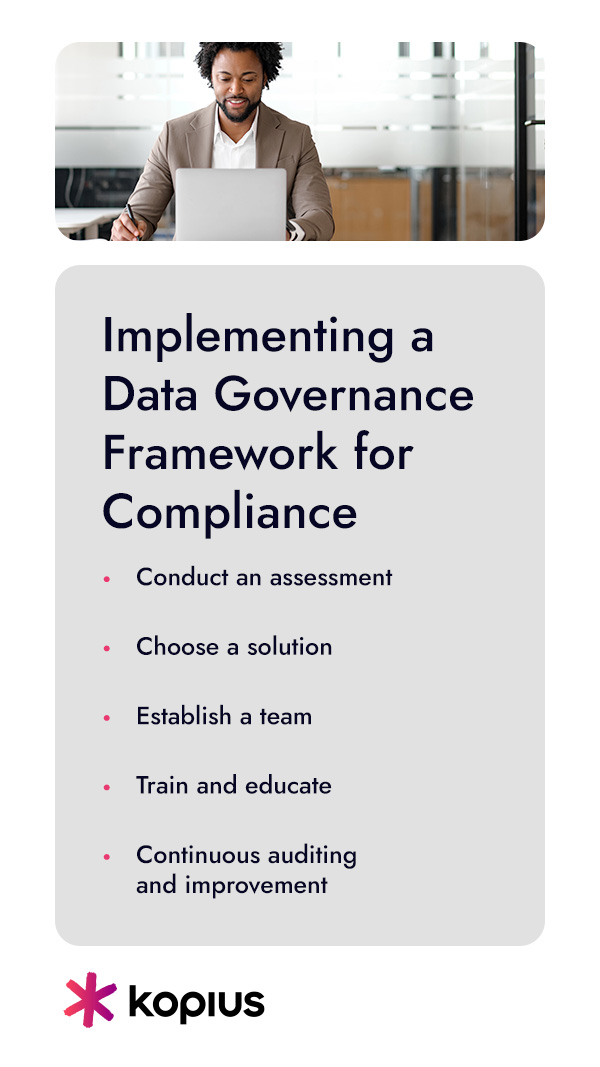
Every organization has unique needs for meeting compliance regulations by state or industry. However, there are some practical steps you can follow for effective data governance implementation:
- Conduct an assessment: The first step is to identify your organization’s data needs. What are the current noncompliance risks you’re facing? Identify and catalog all data assets and determine how they should be handled moving forward.
- Choose a solution: If your organization has vast amounts of data or significant security issues, it’s time to choose a data storage solution or data security compliance service to help you address your data needs.
- Establish a team: Create a data governance team or committee within your organization to help facilitate cross-department collaboration and oversee continuous auditing. This cross-functional team should include compliance, business, legal, and IT team members who routinely develop, improve, and enforce data governance policies.
- Train and educate: Once you’ve developed and documented your data governance policies, it’s critical to make sure all employees understand their role in maintaining data integrity. Provide training on the importance of data governance and compliance to raise awareness of all new and existing policies.
- Continuous auditing and improvement: As with any company-wide adjustments, it’s important to regularly review and update your data governance framework to align with current regulations and arising cybersecurity risks.
JumpStart Your Data Governance and Compliance
Data governance is nonnegotiable, especially when it comes to regulatory compliance. However, aligning data governance with compliance requires careful balance. At Kopius, we offer data security compliance services to help businesses meet their industry standards.
Our experts will manage your data collection and establish an infrastructure that makes compliance fulfillment more achievable. As a reliable data security compliance company, our top goal is to mitigate data security breaches without restricting your business growth. Contact us today to see how we can help you meet your data security obligations and learn about our JumpStart program.
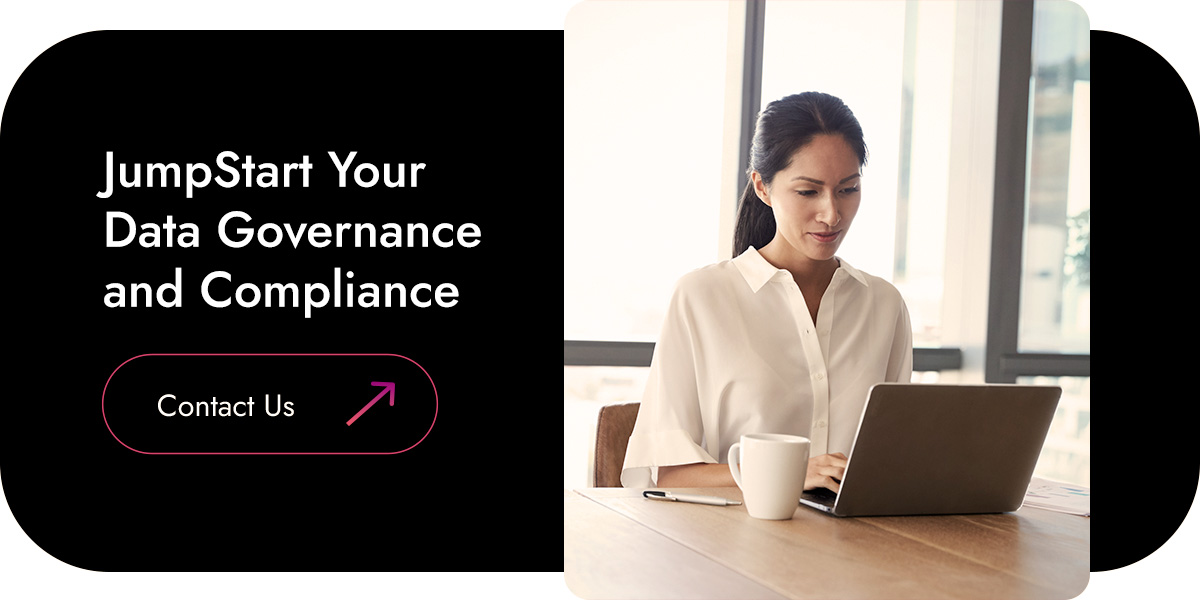
Related Services: